Monday, Oct 11, 2021
The role of AI in helping hospitals forecast and manage patient flow
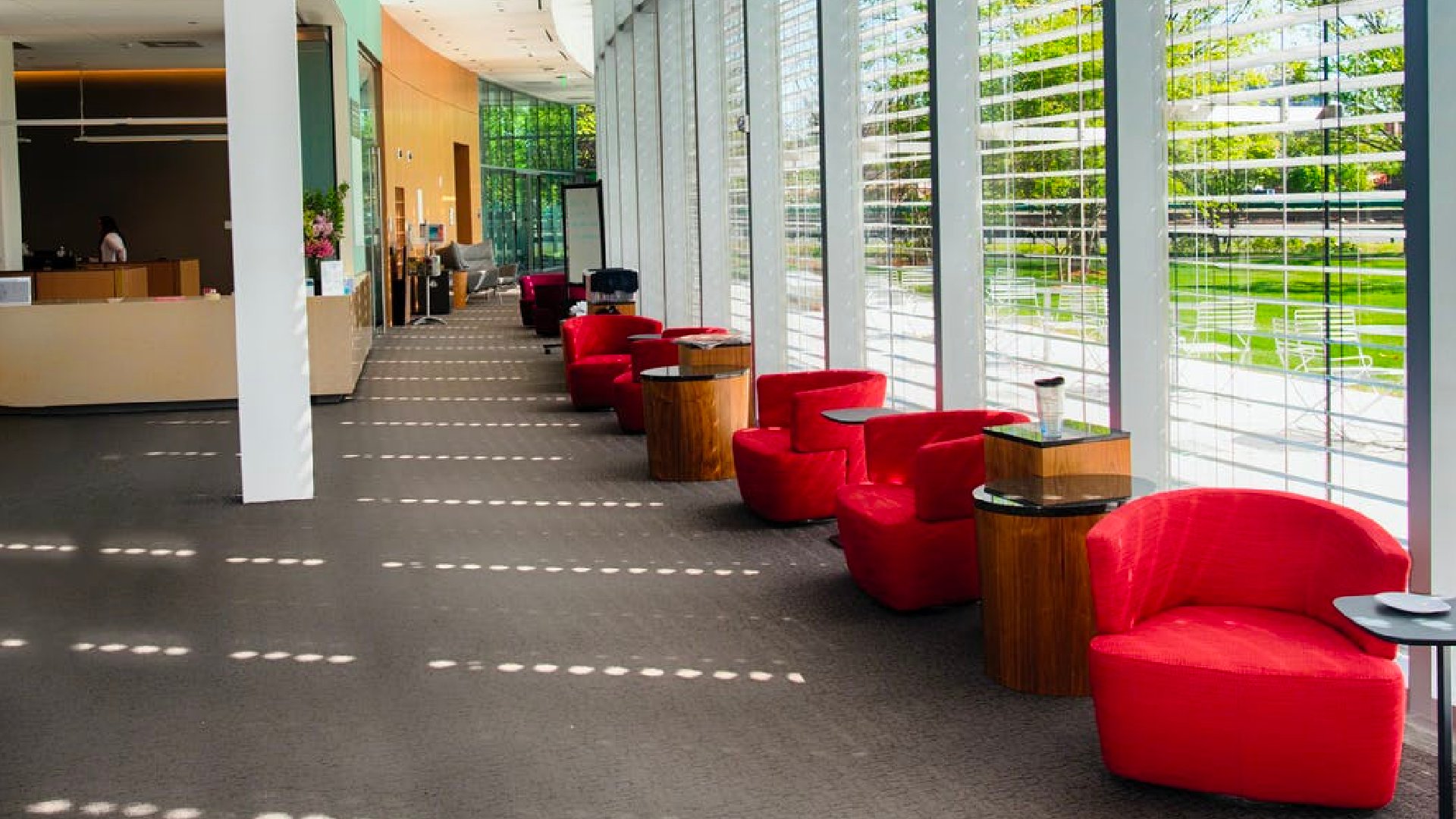
Hospital leaders have faced unexpected surges in patient demand, meaning the ability to anticipate and adapt to rapidly changing circumstances is more important than ever. What if we could predict potential bottlenecks in patient flow in real time – and prevent them before they occur?
Hospitals around the world have long faced challenges with bed and staffing shortages to meet demand for acute care, a situation that has been exacerbated by the pandemic. Across the world, emergency departments (EDs) manage overcrowding even in ordinary times, with intensive care units (ICUs) often operating at or near capacity. The result, all too frequently, is waits and delays, leading to frustration, anxiety, and potentially harmful outcomes in patients, while increasing pressure on staff [1].
While our first reaction may be to assume that more beds and more staff are needed, the real issue is often not a lack of resources per se, but one of patient flow: anticipating and knowing when to transition a patient from one care setting to the next. It’s a dynamic and fast-moving challenge.
Managing patient flow calls for an enterprise-wide view across different parts of the hospital or hospital network, something that is often missing. With clinical and operational data dispersed across disparate systems, care teams lack wider situational awareness beyond their unit or department. This lack of readily available and actionable information can hamper patient prioritization, slow down patient transitions, and lead to unforeseen bottlenecks in patient flow.
The COVID-19 crisis has highlighted many of these challenges, while also encouraging smart thinking around tackling them. Healthcare providers are sharing data in real time to visualize untapped capacity, facilitate patient transfers and to forecast and prepare for future demand. Using the power of AI and predictive modelling, we can extract relevant insights on patient flow and patient care needs from vast amounts of real-time and historical hospital data, to predict capacity needs in the next 24 to 48 hours.
As we move to a post-pandemic era, we have the opportunity to embed these data-driven practices into everyday management of patient flow – from hospital admission all the way to hospital discharge and, ultimately, monitoring in the home.
Managing transitions across care settings with AI
A typical scenario might involve a patient being taken to the hospital with heart palpitations and shortness of breath. While en route, a notification is sent to the patient flow coordinator in a central command center who oversees current and predicted patient capacity across a network of hospitals.
With an instant picture of which hospitals have beds available, the patient can be directed to a hospital where they can swiftly receive the care they need. While the patient is triaged in the ED, care teams can prioritize further clinical evaluation based on, among others, a machine learning algorithm that combines patient vital signs and physiological data to predict risk of health deterioration. And, with an up-to-date overview of bed availability across the hospital, the patient can be pre-allocated a bed in the right care unit, in close alignment with the team on the floor.
When the patient is stabilized in the appropriate critical care unit, patient flow teams can begin to plan the next steps in the care journey. Smart algorithms can help to estimate when the patient will be ready to be transferred to a lower-acuity care setting in the hospital for telemetry monitoring. Based on a transition review list that is updated dynamically, the patient flow coordinator can assist physicians in prioritizing clinical evaluation of patients who may be ready for transfer. Having sight of how many patients in the ED are waiting for an inpatient bed helps identify potential bottlenecks early and manage patient flow accordingly.
Once the patient is deemed stable enough to be transferred to the medical-surgical unit, discharge readiness scores and readmission risk will provide a clear picture of when they are ready to be discharged.
Improving patient flow and reducing length of stay
With thousands of patients flowing through a hospital network on any given day, it’s easy to see the benefits of having centralized clinical and operational insights to manage patient flow.
Rather than working in silos, healthcare providers can coordinate care across their entire enterprise. Coupled with the power of predictive analytics, it becomes possible to manage patient flow proactively from one care setting to the next, preventing congestion and overuse of critical resources.
For patients, it means they don’t need to stay in the hospital any longer than necessary. This, in turn, offers other patients a better chance of getting access to the critical care they need. As a result, healthcare enterprises can serve more patients, thereby reducing backlogs. Smoother patient flow can also benefit their bottom line. For example, one US hospital estimated that they could save $3.9 million annually by eliminating ED overcrowding through accelerated transfers to inpatient settings [2].
Extending care coordination from the hospital to the home
In the future, centralized care coordination could be further extended into the home, using remote diagnostics and monitoring to keep a caring eye on patients as they resume their daily lives. As my colleague Roy Jakobs has outlined before, improving transitions between acute and post-acute care is one of healthcare’s biggest opportunities as it becomes increasingly distributed. Again, this is where predictive analytics in healthcare could make a difference.
Ongoing health monitoring at home has already demonstrated its value in managing chronic diseases such as COPD, with one pilot study in the US showing an 80% reduction in acute 30-day COPD readmissions and $1.3 million in savings [3].
Ultimately, that is how future healthcare systems will manage patient flow more effectively and efficiently across the patient journey – relying on data-driven decisions to ensure that the right patient gets the right care in the right place at the right moment. With greater understanding of patterns in patient demand and patient flow, healthcare leaders can also form long-term strategies for hospital capacity planning, making better informed decisions about the types of care settings and resources that are needed.
Uncertainty will remain a part of healthcare, as it is in life. But by better forecasting what may happen next, healthcare systems will become more adaptive and resilient in the face of change and crisis. That’s a prediction I’m willing to make.
References
[1] Rutherford PA, Anderson A, Kotagal UR, Luther K, Provost LP, Ryckman FC, Taylor J. Achieving Hospital-wide Patient Flow (Second Edition). IHI White Paper. Boston, Massachusetts: Institute for Healthcare Improvement; 2020. http://www.ihi.org/resources/Pages/IHIWhitePapers/Achieving-Hospital-wide-Patient-Flow.aspx
[2] Foley M, Kifaieh N, Mallon W. Financial Impact of Emergency Department Crowding. Western Journal of Emergency Medicine, Volume XII, no. 2: May 2011, 192-197. https://pubmed.ncbi.nlm.nih.gov/30193738/
[3] Reducing hospital readmissions with integrated COPD care. Philips case study. [Results are specific to the institution where they were obtained and may not reflect the results achievable at other institutions.] https://www.philips.com/a-w/about/news/archive/case-studies/20191001-reducing-hospital-readmissions-with-integrated-copd-care.html
You May Also Enjoy
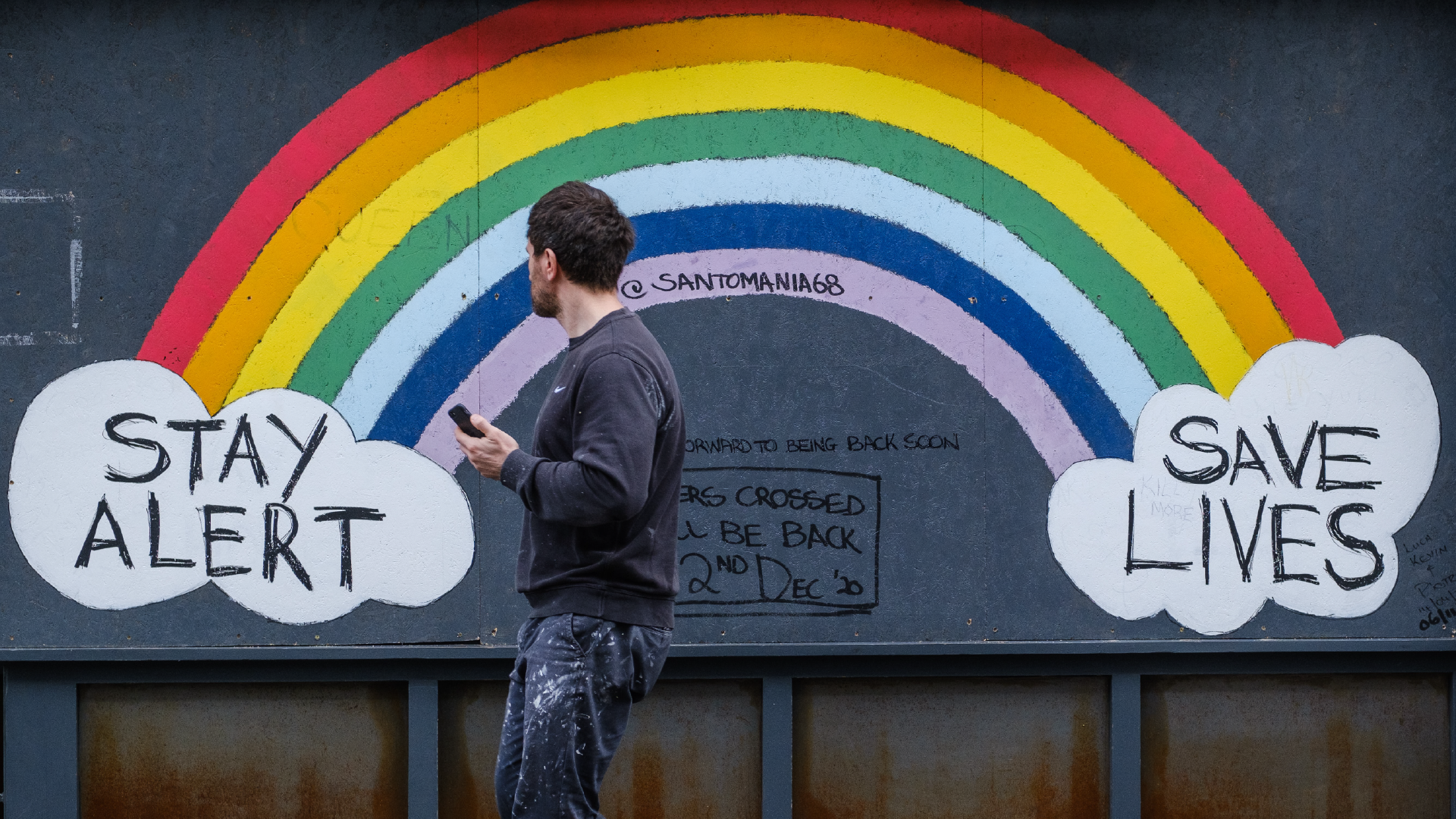
Wednesday, Apr 15, 2020
COVID-19 Updates with David Van Sickle
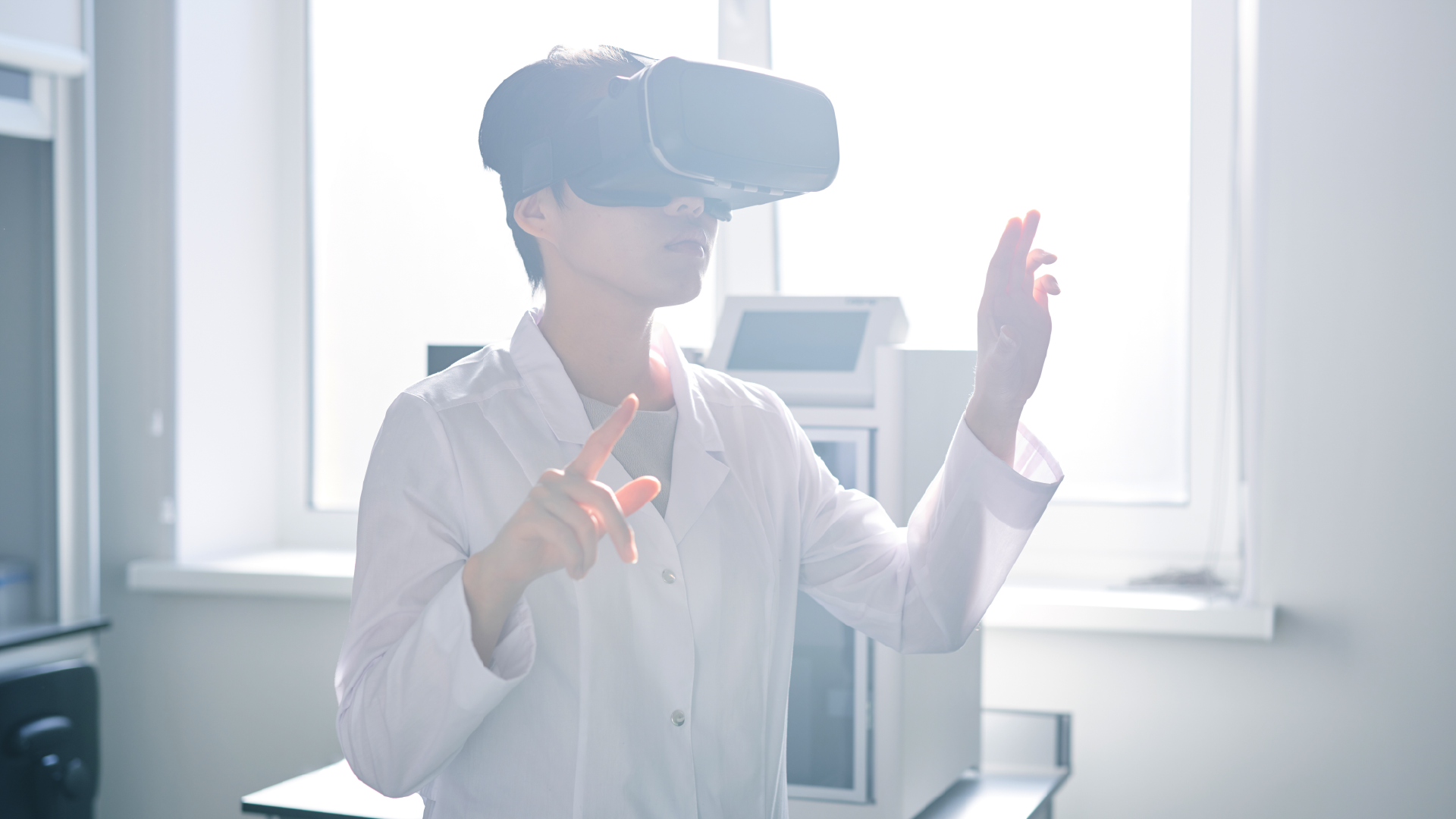
Friday, Oct 9, 2020
Navigating 2020 by Embracing Innovation
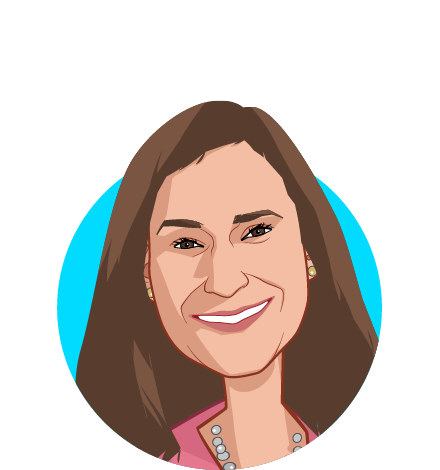
Roberta L. Schwartz
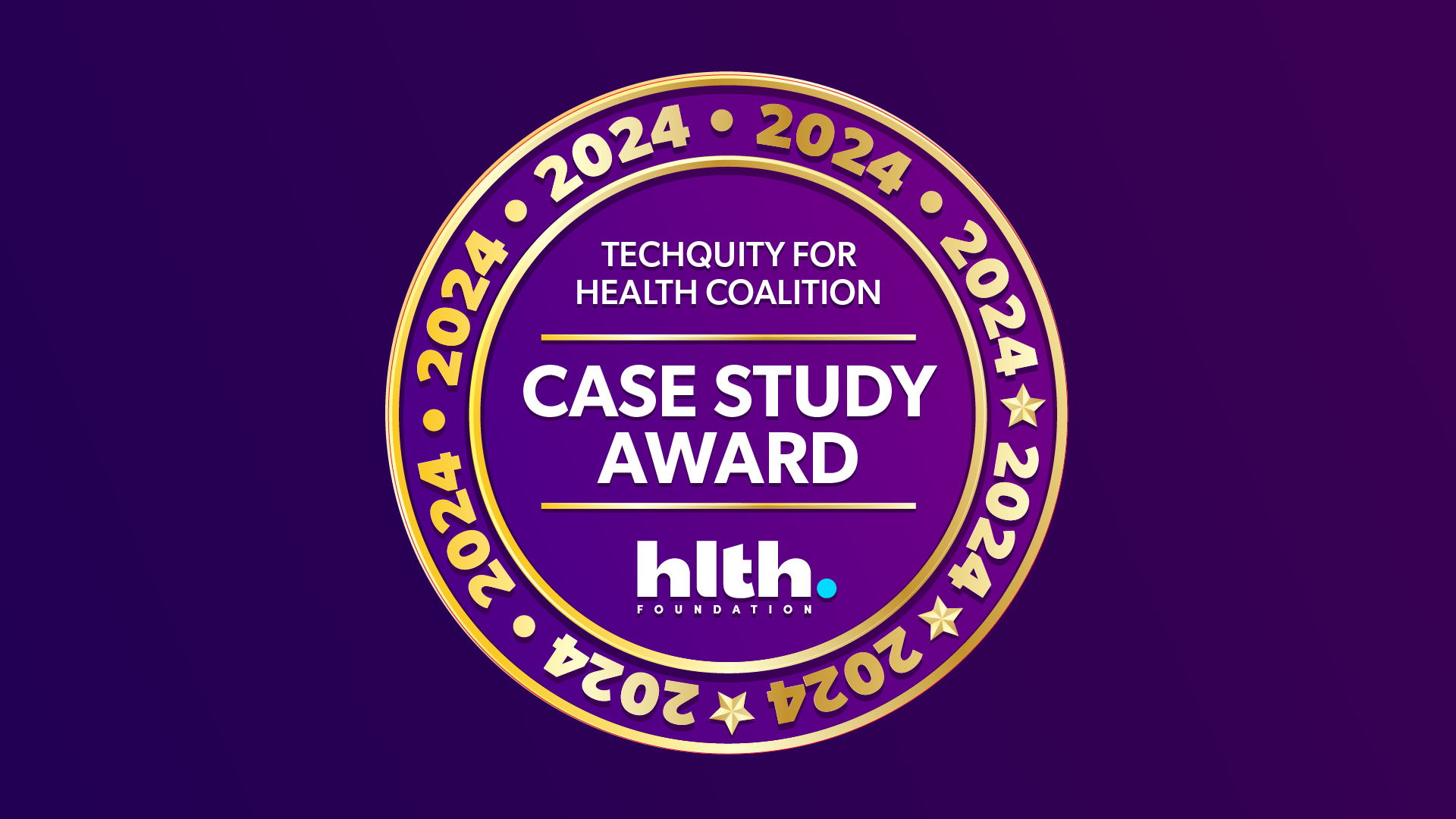
Tuesday, Feb 27, 2024
First Techquity for Health Case Study Competition Winners Announced: A Groundswell of Action on Health Equity